3 Factors Dominate the Success or Failure of All Conversational AI Experiences
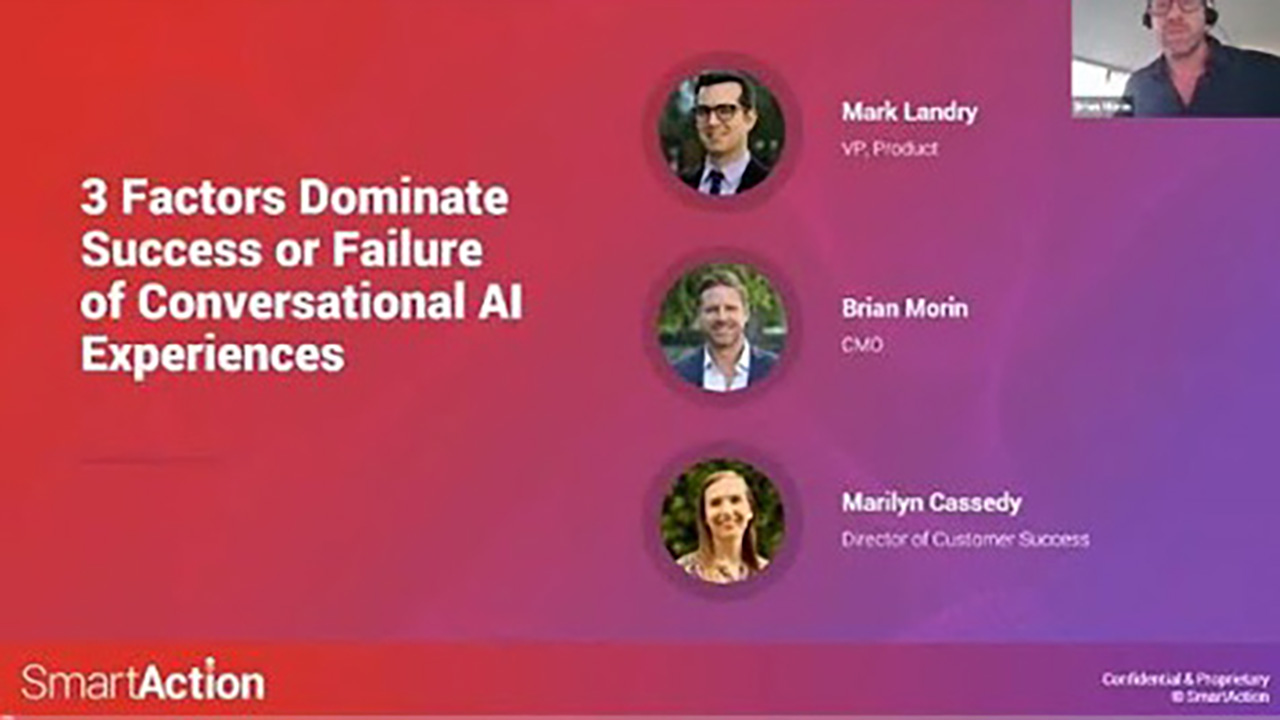
When it comes to delivering award-winning AI-powered CX that outperforms live agents over voice, success or failure comes down to 3 core factors that very few seasoned contact center leaders understand very well.
Don’t miss our most densely packed informational webinar to share never-before seen insights.
Key Takeaways:
- Why is speech rec over telephony such a challenge & how do vendors differ on approach
- When & where to use the right speech rec engine (i.e. Google/Amazon vs. domain-specific ML engines tuned for specific grammars)
- The art of CX design & makeover examples of why it matters
- The process of iterating over time through monitoring & reporting
Watch This
Webinar
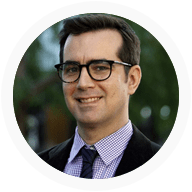
Mark Landry
VP of Product,
SmartAction
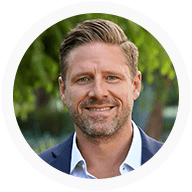
Brian Morin
Chief Marketing Officer,
SmartAction
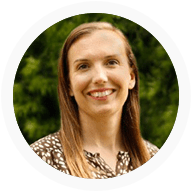
Marilyn Cassedy
Director of Customer Success,
SmartAction
3 Factors Dominate the Success or Failure of All Conversational AI Experiences
On-Demand Webinar
Brian Morin: Well, good afternoon, everyone. I hope you can hear me. My name is Brian Morin. I’m your host moderator today sharing some of the content. With me is Mark Landry, a VP in product. He also oversees our human-centric CX design team. Then also with us is Marilyn Cassedy, our director of customer success. Both of them are involved in CX disciplines when it comes to managing the AI powered CX. Today, we are chatting about the three factors that dominate success or failure of conversational AI experiences. We have done here at SmartAction more than 100 deployments of conversational AI over voice or voice plus chat or voice plus chat plus text. It is an omni-channel experience that we deliver, but we’re able to speak from that with the breadth of that experience. I should be very quick to point out Marilyn will probably be the first to tell me, “Brian, there’s certainly more than three.”
And there are more than three, but when we boil the ocean of the most important factors involved in delivering a great your voice experience with AI, and we take it down to its very essence, there are three fundamental areas that you cannot get wrong. If you get any of these three areas wrong, it just doesn’t even matter what else you’re doing that’s right. From that standpoint, we are going to start with the AI piece of this conversation as it comes to speech rec, and then we will dive into a couple of the other very important aspects like the art of CX design and the inner process of improvement that involves big data tools, monitoring and reporting and how you can chase that frictionless experience over time. If you’re joining us today and by chance you don’t have any familiarity with SmartAction, I can make this just super fast.
We do AI-powered virtual agents omni-channel and we do it as a service. What that means is that we deliver the full conversational AI technology stack. It’s turnkey, it’s omni-channel that starts in voice, and then we skilled digitally for customers to chat and text. We’re not just a software company throwing software licenses and seats over the fence and wishing you good luck on your journey. One of our unique differentiators is that we actually bundle the technology with end-to-end CX services, and that means everything, the design, the build, the ongoing operation. That’s simply because Omni-channel CX is really hard, and it does take a team of experts to do that across a variety of disciplines. We’ll share a little bit more about that later. If all you’re doing is just a chat bot and you’re just trying to stand up a chat bot, you can likely do that on your own on a DIY platform, but the moment you include voice and you’re including other channels, there’s a lot more complexity involved.
That’s why our customers prefer to use this as a service. We operate and manage the AI-powered CX for more than 100 brands, and we’d like to think our approach is working for us as we are the top rated solution on Gartner Peer Insights as rated by customer reviews of 4.8 out of five. Did I say all of that fast enough? If I haven’t said enough about SmartAction already, we would say don’t take our word for it. You can look us up on our website. You can see 18 published case studies and some of the accolades along the way. To dive into just a little bit about what we’re talking today, and I should mention we do have set content, but please we’d like this to be as audience driven as much as possible, so you can participate just by using your chat box or your Q and A box to have a comment or question. We’ll handle what we can as we go along, and then we’ll move to a full Q and A at the bottom of the hour to answer anything that we’ve missed.
We’ll start here in this conversation on voice. The focus on this conversation in this webinar is over voice, although we do provide experiences over other channels. A lot of that has to do with the fact that it’s just because the voice channel is the hardest to deliver a great experience. We’ve all had that experience. Why is it that most voice implementations deliver bad experience? If we were to say it in three words, it’s because it’s hard. Now, what we want you to do is we want you to imagine a different kind of an experience, an experience that is personalized, an experience that’s predictive, an experience where everything happens in natural language with AI that sounds like the human, has the ability to read and record data like a human, take cognitive action like a human. I’m going to tee up Mark right after this slide actually to just give you a live example, do a live demo, what that sounds like, but you can just see the Tweets from real customers on screen interacting with our AI that are our clients like to share with us, like understood full sentences, the most painless experience I’ve had, better and faster than humans.
One guy, he even goes on to marvel about every single step in the process. During his AAA emergency roadside assistance, when he called in, he was expecting to be helped by a human, but instead he was helped by our AI and marveled, so kind of cool. Rather than talk about it, Mark, why don’t we play a game of show and tell. Mark is in the final stages of delivering this particular application to some auto dealerships, and I believe that we are the very first in the industry to deliver a conversational omni-channel conversational AI experience to auto dealerships. I think it’s someone we can all identify with, calling in to schedule an appointment or some kind of service. Mark, is there any setup you need to give here before kick-starting the demo?
Mark Landry: Sure. Thanks, Brian. You guys can hear me okay, Brian? Okay, great. This is Morrie’s. This is a set of dealerships in the Midwest. They have their application going through our UAT process right now, so user acceptance testing. We’ve built it, we’ve QAed it and now they’re going through and making sure that’s what they wanted for their customers in terms of an experience. What I’ll do, this is a UAT environment. It’s active, it’s close to production. It’s pinging Morrie’s database for available times for the oil change that I’m going to try to schedule. What you’ll see is that negotiation, it’ll be a human voice conversation. You’ll hear the app sounds like a human, but it doesn’t pretend that it’s human. It’s not trying to fool you. You can tell it’s an IVA. Okay. With that, I’ll go ahead and just call them up.
IVA: Good afternoon, and thank you for calling Morrie’s Cadillac service department. Am I speaking with Mark?
Mark Landry: Yes.
IVA: Are you calling about your appointment scheduled for Saturday, October 24th, 10:45 AM?
Mark Landry: No.
IVA: Okay. Would you like to schedule a new service appointment?
Mark Landry: Yes, I would.
IVA: Do you need an appointment for general maintenance or do you need repairs?
Mark Landry: General maintenance.
IVA: Great. Now, let’s set up your service. Please tell me if you need an oil change, tire service, a status update or something else.
Mark Landry: Oil change.
IVA: Got it. Will you be needing additional services for your vehicle?
Mark Landry: No, thank you.
IVA: Are we talking about your 2018 Honda Civic?
Mark Landry: Yes.
IVA: Thanks. Please tell me the approximate mileage on the vehicle. You can also say, I don’t know it.
Mark Landry: 30,000.
IVA: Our shuttle service operates within 10 miles of the dealership. Are you planning on using our shuttle service or would you prefer to wait?
Mark Landry: I’ll wait.
IVA: Okay. One moment while I look up some account information. The first available appointment I have for you is Thursday, October 22nd at 1:15 PM. Will that work for you?
Mark Landry: No.
IVA: Do you have a preferred day and time you’d like to schedule your appointment?
Mark Landry: October 24th, 10:00 AM.
IVA: I don’t have that exact time, but I do have Saturday, October 24th at 12:15 PM. Will work for you?
Mark Landry: Yes.
IVA: You’re all set. Your appointment has been scheduled for Saturday, October 24th at 12:15 PM. Your confirmation number is X05G3W18ZP. With your permission, I’ll text you a confirmation and any updates for your appointment. Standard data rates apply. Would you like the confirmation texted to you?
Mark Landry: Yes, I would.
IVA: Would you like to receive the text messages at the number ending in 4994?
Mark Landry: Yes, please.
IVA: Great. I’ll send you the confirmation within the next few minutes.
Mark Landry: I got the text already. It says, “Hi, Mark. Your appointment at Morrie’s Cadillac is all set for Saturday 10/24 at 12:15 PM for your 2018 Honda Civic. We’ll text you a reminder the day before the appointment as well. Thank you for being a valued Morrie’s customer.”
Brian Morin: A couple of things that we should point out here, Mark, is one, I know that when it came to scheduling or negotiating the time, you gave a date knowing the exact date, but you could have said like next Friday, you could have used words like noon or morning and would have had the correct type of negotiation back.
Mark Landry: That’s right.
Brian Morin: You were calling, the system already had you on file, so you were already in the CRM. What about instances if you were to call in and you were a new caller, how would that experience have been different?
Mark Landry: Yeah, good question. If I were a new caller, I had never been to the dealership before, they didn’t have me on record, what we do is we work with the dealership to say, what do you need from this person in order to make the appointment? Most dealerships that we’ve dealt with say, “Well, we just need their name, first and last name and phone number, and then the make, model of their car, make model and year actually.” We’ll get the person and the car on file and set the appointment. From then on, that account is in their CRM.
Brian Morin: Gotcha. Thanks, Mark.
Mark Landry: Thank you.
Brian Morin: If anyone wants to go a little deeper on that and see what those other experiences look like with different names or vehicle, different year, make and model and vehicle capture, happy to step you through them. If we were to jump into the first core factor, the first core factor of a great voice experience is centered on what AI is doing in the area of speech recognition. What we are talking about today, I want to make this very clear, we’re talking about conversational AI that’s purpose-built for a telephony and purpose-built for limited grammar use cases. I will explain in a minute why that’s so important to having a good voice experience. Speech rec over telephony is really hard to do well, and that might not surprise anybody considering the poor experiences we’ve all had, but you may not necessarily understand why and what the bleeding edge of AI is doing to solve it.
It’s very different than speaking directly into your phone or your home device, which is a high def experience as long as it’s connected to the internet. That’s why speech rec directly on your phone or your home device is so good because you’re capturing all the highs and lows at the device level, which makes it easy to distinguish utterances that can relate those to letters, syllables, words. The moment that you call into a customer service line and those sound waves travel over an outdated telephony infrastructure, the resolution is reduced significantly to 8K in most cases. So it’s cutting out more than half the highs and lows in half, and if that wasn’t bad enough, it adds noise. This is why conversational AI over telephony is a different animal, and it represents its own difficult challenge. It’s also why the same transcription-based engines like Google or Amazon, they just don’t deliver a good enough experience at the contact center level because they are now 50% and less accurate.
You have to have AI that’s purpose-built for this kind of challenge. Now, I will note in a minute, we have found use cases where Google will outperform in certain customer service use cases and I’ll explain where and why in a minute, but let’s explain the type of approach that you have to take to get the best experience as far as capturing over voice. It’s very different than a transcription-based engine approach that might use CUs by like a Google or Amazon. They have to be all things to all people and transcribe every utterance according to a statistical based confidence score, and what we need is we need an AI engine that’s number one, it’s trained on domain specific telephony audio, but number two, and this is what’s most important is that it can be fine tuned for specific questions that have specific answers.
In customer service, most questions have a limited number of grammars that need to be accounted for, and so if you know what those grammars are, you can narrow the aperture of what you’re listening for to just those grammars or anything that sounds even remotely similar to one of those grammars. If you ask, let’s say like a yes, no question, you already know the only outcome is yes or no. That enables you to be predictive, and that’s something that you can’t do with a transcription-based service like a Google or Amazon. This allows you to manually tweak how you weight your language acoustic models against that very question against what you are predicting to hearing. We’re going a little deep in the weeds, but there’s almost no other easier way to explain it. I’ll be the very first to admit that this approach is not a very scalable approach because this means that a lot of custom work is involved because you are tuning acoustic models for every single question that’s asked in a given application.
It’s also why you can take up to eight weeks in a build process if we are accounting for every possible utterance to any given question that we’ve never tuned before because then you have to manually tune it. Now, the good news to that, at least in our case, we are now supporting over 100 customers, more than 100 different use cases across almost every industry, so it’s becoming rare and rare for us to run into an interaction that we haven’t automated already. That means that we’ve likely already done this heavy lifting. For any new customer that comes to us, we can get them live faster as a result. While the initial build to a new application can be a little arduous customizing for specific questions and specific answers, it really is the only approach that can deliver a good experience over telephony.
It means why we can have such a high accuracy rate as we do, and it also means that the resulting takeaway is that the more that you widen the scope and the more that you widen the aperture of what you’re listening for, that means the less accurate this approach becomes. If the scope gets too wide, well then in those cases, we will opt for a Google transcription style service because that will outperform in those use cases. We’re not by any means married to using this approach. We are a CX company and at the end of the day, we’re going to use whatever delivers the best experience, but ultimately it comes down to choosing the best tool and the best customer experience that is with use cases, and most of the time that is with narrow aperture or a narrow scope that we can pattern match again. Let me just give one real world example of this.
I know on the previous side I talked about a very narrow aperture. I’m talking about here, a use case where we do address capture. Now, address capture is a really wide aperture, and we do address capture for a lot of clients like designer shoe warehouse, choice hotels, just to name a couple, but the only reason and the reason we can do it really well is because we can pattern match against street names as long as we know the ZIP code. You can see on the screen as soon as we get a ZIP code from a customer, we can do a data dip and we can pull up all the street names that we can match against. This is what gives us that really high accuracy rate. If we couldn’t pattern match, then we would have the inability to predict. Without that ability, we would default to a transcription-based engine like Google for something like this.
We do alphanumeric capture for certain use cases, and we are the only company in the industry doing alphanumeric capture over telephony. We do it for model names and serial numbers, for product registrations like with Electrolux, the second largest appliance manufacturer. We do it for VIN numbers like Honda, we do it for state foreign policy numbers and insurance. We don’t like alphanumeric capture, unless there’s a defined scope or pattern we can match against. The cases I’ve mentioned are ones where we’re not looking for all the letters in the alphabet. There is a sequence, so we’re only looking for select ones, and that gives us the ability to weight whatever we hear against what we know that we’re listening for. We do the capture of makes and models on vehicles like you just heard, and for AAA emergency roadside assistance.
That’s not a narrow scope. The aperture makes and models is really wide, you would think that you would need a transcription-based engine, which frankly wouldn’t work that well, but since we can pattern match against the database and make some models, we can do it far better than you can get from a transcription-based engine like a Google. I think that I’m running a little long on time. I’m not going to cover this slide. This is just to say you have to choose ultimately the right speech rec for the job. This is a case where if you have language models or those acoustic models are similar, a transcription-based approach would work better in that use case. So it’s just about finding the right tool. Mark, I’m going to transition over to you and I want to tee this up.
For those that are listening in, if you can see on the screen, when we step beyond the technology, we have to step into a conversation, into the humans that are actually required to run the AI and open up this black box a little bit. If you see on the screen, if you attempt to do voice automation on your own, particularly natural language automation, the outer ring is all the jobs or functions, you need experts in their field doing that role. Ultimately, kind of said teed up at the beginning of the call, this is why we deliver our technology as a service because of the complexity involved in delivering a great voice experience. Mark, with that said, a lot of this begins first with human-centric CX design. We know when we’re talking to somebody for the first time the full art of this isn’t fully appreciated until we actually stepped through the sausage making process with them. Why don’t you give us just a quick high level overview.
Mark Landry: Yeah, sure. Thanks. I think that it’s very likely that people don’t understand what goes into making an AI conversation seamless as a customer experience. You see the 18 members here, these are eight different departments within SmartAction that go into building an application, building a customer experience for your customers. We don’t just build it and set it and forget it, we take care of it through the life of the engagement with you. We mature it, we tune it. We add modules as your needs change, et cetera. We don’t have time to focus on every single one of these eight disciplines today, so I’ll focus on what I’m responsible for, which is the customer experience, design and strategy. What my team does is we design the human experience with as little friction as possible, and there is an art to that. If you don’t have good design, you’re going to have a bad design.
There’s going to be a design, no matter whether you do it well or you don’t. What we found for increasing that containment and the user acceptance of the AI is to design with empathy. What I mean by that is that we know as human beings, we can’t be on every automated call, but our designs will be and if we can infuse those designs with an empathetic approach to the person who’s calling in. We’ve been there. We’ve called in customer service. Why do we do that? Because there’s always some issue. We’re not calling in just to say, “Hey, you guys are doing a great job.” How many times do you ever do that in your life? You’re missing an order, something that you got is broken, your TV’s not working, you’re curious about your account, you need to add somebody to your insurance. You need to do something. You have a goal that needs to be accomplished, but more than that, you have an emotional tension when you start this call.
Our job in terms of designing with empathy is to take that ball of tension and relieve it as soon as possible within the phone call. If we can do that within the first 15 seconds, if we can say, “Hey, Mark. I understand that you have an issue. Can you tell me what the problem is?” We have asked that person for what their tension is, to describe your tension for me. Then we understand that use case and say, “No problem, I’m going to help you.” Within 15 seconds, with our client’s business rules, we can get away with that. If we go on to the next slide here, Brian. This is an example that we did for AAA. What we did was we took a look at their IVA, basically their automated system. It had a robotic voice, it interrogated the caller for a minute and a half, give me your membership number, your name, your ZIP code, all these things. It took without giving.
It never gave that person a sense of I’m going to take care of you. It’s going to be okay. You’re stranded on the side of the road, I understand. We’re going to get you moving again. Don’t worry about it. It doesn’t take much to relieve that tension. You don’t have to invest billions of dollars in an emotionally intelligent machine. We’re emotionally intelligent. We’re putting our intelligence into that design. Interrogating their members, the roadside assistance company was designing their product for their needs and not the member’s needs, just getting data, ignoring the caller’s feelings. It didn’t ask him what’s wrong until a minute and a half. This was a case where this guy, Alex was on the side of the road with a dead battery and he and his wife, Michelle needed to get their daughter home to take her medicine within an hour. They didn’t know how long it was going to take to get their battery jumped or replaced or whatever it needed, so we took that and did a complete makeover on the conversation.
Brian Morin: Mark, [crosstalk 00:24:51] do we want to play the makeover example or?
Mark Landry: Sure.
Brian Morin: Let me see where we’re at on time.
Mark Landry: I’ve basically described the bad one. I mean, if you want to play the first few seconds just to give an example of.
Brian Morin: Yeah, it might be a little arduous for the audience to sit through the whole thing because it is a little long, but yeah, let’s do that. Let’s play the beginning.
Mark Landry: Just so you can see what it sounds.
Ursa: Thank you for calling AAA. My name is Ursa, and I’ll be your roadside service assistant today. Are you in a safe location?
Customer: Yes.
Ursa: I see the phone number you are calling from is (916) 868-3359. Is this the best number to reach you [crosstalk 00:25:34]? Please say or key in this whole 16 digits that appear on the front of-
Mark Landry: Okay, that’s enough.
Brian Morin: It’s painful.
Mark Landry: Very painful.
Brian Morin: It’s already painful.
Mark Landry: It is.
Brian Morin: Yeah.
Mark Landry: It’s a super long call.
Brian Morin: My attention went up listening to it, so five is that caller.
Mark Landry: Right.
Brian Morin: Yeah.
Mark Landry: In the redo, in the makeover, we said, “This call doesn’t need to be two, two and a half minutes long. We understand, by the phone number, the person. We know who they are because we can dip into the database. We don’t need to ask for their full 16 digit member number.” Just give the guy some sympathy upfront and then take care of the problem, and then you’re good to go. We can play this. We’ll see that within 15 seconds, we ask them what’s wrong.
Brian Morin: Well, and I think the key takeaway here, Mark, is that the makeover that you did, the entirety of the call took less time than it took the first call to even ask the problem.
Mark Landry: That is the key.
IVA: Thank you for calling AAA. I’m sorry you’re having trouble. Are you in a safe location?
Customer: Yes.
IVA: Okay, let’s get you moving again. What seems to be the problem with your vehicle?
Customer: I need a jump.
IVA: No problem. We can test, diagnose and replace back-
Brian Morin: I don’t think we need to list the whole thing. I think the audience could see the tenor of the difference, but the case in point being for the audience is that everyone can have the same set of AI tools, but not have the same outcome on the customer experience. This is just to showcase what a difference it makes to go in deep on your CX design. Thanks, Mark.
Mark Landry: Thank you.
Brian Morin: Marilyn, I’m going to jump over to you. This is one big area when it comes to conversational AI that most contact centers miss because they’re just haven’t been familiar with this process before. They’re used to a world of an IVR where it’s design, build and you’re done, and its first day is the best day it ever had. Conversational AI is very different. You’re trying to have conversations with machines. This is enormously complex. In fact, day one is not when you’re done, day one is actually when you’re just beginning your conversational AI journey because you and your team, your whole job and engagement is about chasing that process of improvement through iterating and improving over time.
Marilyn Cassedy: Absolutely. I think that you’ve hit the nail on the head, right? We pay a lot of attention, and in putting something like this together, you should pay a lot of attention to the reporting that you’re going to get out of the application because that’s how you’re going to determine what you want to iterate against. There’s a couple of different aspects to that, that I think this conversation makes the most sense to start with a few definitions and then we can get into how we use that data-
Brian Morin: Yeah, good point [crosstalk 00:28:19].
Marilyn Cassedy: When we’re thinking about how do we get insight out of this application, you need to think about your toolset, right? The starting point is got to be with the data. I think that tools like Splunk, Power BI, other similar kinds of really enterprise software are a great starting point for the conversation. From these, you’re really going to gain a couple of different kinds of information. On the Splunk side, it’s much more of a monitoring is everything working as we expected, are the backend connections working correctly. Then on the Power BI side, we’re going to learn more about the callers, the users themselves, are we getting more elite numbers in the system and we are regular members, what do we need to do to make sure that they’re having the best possible experience? So it’s really starting with your data selection and your data subsisting tools as a beginning for understanding what’s even happening there.
Beyond that, then we actually would recommend breaking your reporting down into a few different sort of structural pieces, and each of these is going to give you different kinds of information that’s going to allow you to continue to consider the caller experience and continue to make improvements. These three different reporting types are really increasing order of details. So an outcome is just how did the call wind up? Was it someone who abandoned the call halfway through, is this someone who’s completed the call successfully and is happy with where they wound up or has achieved what they needed to, that really, really high level. Endstates on the other hand are going to talk about where the caller wound up in a more granular way. While a finish for an outcome means that that caller might have successfully completed the call flow, endstate is going to tell you where they wound up.
Did they successfully book a reservation, did they successfully cancel a reservation? It’s important for us to know what the customer did while they were going through in order to better refine their experience. Really both of those lead us to breadcrumbs, and breadcrumbs I think you’ve got to go back to sort of Hansel and Gretel and your storybook days here and think about a trail of breadcrumbs that are going to allow you to follow the caller prompt by prompt through a system. With breadcrumbs is where we really unlock some amazing potential in terms of tuning an application and understanding where is their friction, where did we think that we would be able to ask a question when we were considering their design process that really customers aren’t prepared for it. So this is a huge part of the value of the reporting process.
Brian, if you want to go to the next slide. We can take a look at how then these breadcrumbs and other types of reporting can help us. In this example, we’re just looking at trends over time and thinking about what’s happening during these periods that’s making these fluctuate. This specific example is with a retailer where we’re looking at their containment over time versus the number of minutes spent in the phone call over time. If any of you are in retail or if you know anyone who is, you may know that the fourth quarter is quite a busy time for retailers, and actually their call centers by extension. So it makes sense that in December we see a little bit of a spike in both the number of minutes from the phone call as well as the number of contained calls. Maybe their queue times are longer, and so folks are spending more time in the automated system because they know they can get in, get what they need and get out without having to wait on hold.
It’s important then that with those kinds of data points, we use a combination of endstates, outcomes and breadcrumbs to really start categorizing our callers into these five different areas, and these are just example areas. You may have a different business case that requires a different examination of it, but these are some of the high level ways that I think we can define them and that we can use to sort of explore what needs to be done. The two that I would highlight for this team and the place where you’re going to find the most insight are going to be in the virtual agent resistant and the suboptimal experience. Virtual agent resistant, there’s not a ton we can do other than approaching the design with empathy.
If we think back to the AAA example, they might be virtual agent resistant because the virtual agent is interrogating them about their membership number when really they need a jump for their car. This is a great place for us to think about those kinds of quality of life improvements. Then a suboptimal experience, these are folks who have encountered some of that friction in the call flow that I mentioned that the breadcrumbs and our understanding of where they wound up and how the call transferred maybe or ended without a resolution, this is how we can dig in and try to find out more about where we can make those tuning enhancements. It’s really the tuning enhancements that are literally the key to this.
All of the information we’ve shared up to this point is based on the idea of defining some of the terms, and it’s this combination of endstates and outcomes that are going to allow us to highlight that suboptimal user experience and zoom in on it and make decisions about how we can continue to improve the application over time. You can see here that rate them against each other, we’re able to determine exactly which those phone calls are, so that we’re not just listening to all of the phone calls that are hitting an application, we’re listening to the right phone calls where we can gain the most insight when that is necessary. Here’s a great example though of where breadcrumbs can really help us because breadcrumbs are going to allow us to understand. So these are all calls that were abandoned before they reached the end of the flow, and breadcrumbs allow us to understand where in that flow callers abandoned it.
So did they get all the way through the city area? No, you can see that many of the callers are abandoning right when they’re being asked, how many rooms do you want to book? So we learned something there. Is number of rooms maybe not the best place for us to start. Why are we asking for the number of rooms first? Is that how an agent would start this conversation? This is the kind of data that we need to get from our application in order to decide what makes the most sense in terms of optimizing it. In the end though, a lot of this really does come back to ROI, so the ability for us to dig into each of those pieces and each of the different areas where there may be friction to help keep callers in the flow when it makes the most sense is what’s going to ultimately to performance and then of course the ROI.
Even seeing this example, this is that same retailer from earlier in the presentation, and we’re able to basically break down their calls and say that 91% of callers are engaging with IVA either partially or completely self-served during the period that we reviewed here. When we look at the total calls and the number of deflected calls, we’re able to, with data from their call center based on their cost per call, determine what the exact ROI is on the application. Now, your mileage may vary and depending on what kind of design decisions we make, the numbers that you see here may reflect a very conservative case for you or they may be the most optimistic version, but I think it’s really important to understand that the tuning of the application does contribute to this ROI and our ability to have the automated system prove out the purpose that we had for it when we were discussing designing and building it.
Brian Morin: Excellent. Perfect. I had some feedback for a minute.
Marilyn Cassedy: We’ve got you now.
Brian Morin: Marilyn, maybe if you can mute out, I don’t know if it’s being captured on your system. I know we ran just a few minutes past our bottom of the hour time post. We are going to be moving over to Q and A as you can see on screen as far as anyone that’s listening in interested in next steps. You can see the email on screen info@smartaction.com. You can reach out to us. We’re happy to give an engagement. Most often, that starts with sharing a demo experience of what we’re already doing for other adjacent players to you in your own industry or vertical, and so you can see what we’re already doing in your space. We’re likely already supporting somebody.
If it’s beyond that, it’s just sitting down doing a free consultation, getting into some of the things that Marilyn described to find out is your organization ready for AI, are you meeting the right thresholds for fit for AI across some of your different interactions as we dive into volumes and the behavioral characteristics of those. So a lot of questions came in. I will say that those that have a hard stop, we will be sending over a copy of the deck. That’s one of the questions that came in as soon as this is over. As soon as this renders, we will send you an on-demand version of this. It will come from somebody on our team. You’ll likely receive it tomorrow, sometime tomorrow morning, so you can share that with stakeholders if needed. With that said, Mark, I know that there were a number of questions that came in. Looks that you were handling some of these as they were coming through in real time. I think some of these questions make sense for the broader audience, and not sure if you see some of these where you want to start.
Mark Landry: Yeah, thanks. One of the really good questions we had, first off was do you find name capture challenging? The answer to that is yes, we did until we came up with a new way to do it, which was just to ask the person to spell the first name, ask the person spell the last name, and we’re getting in the 90s of percent of accuracy on that. So spell your first name and spell your last name, and then it says, “Oh, did you say Mark Landry?” I did. It’s pretty amazing how that just changed the game on that for us.
Brian Morin: Yup, that allows somebody to capture clean data into their CRM directly from voice.
Mark Landry: Yeah. Another good question was I heard oil change, is that correct was suggested as maybe a confirmation that could have been asked in the demo by the bot, and where do we draw the line on asking a confirmation or just accepting what the person said? Honestly, it goes down to tuning each question. There are certain questions where we absolutely have to ask a confirmation, like if we’re taking a new customer, if I hadn’t been already in the CRM for Morrie’s and the bot was going to take me in as a customer, it would have confirmed my name, my phone number. If it needed to ask my address, it would have confirmed the address. So all the really crucial details that need to be confirmed will get confirmed. Also, if a couple of intents sound similar, we’ll ask the confirmation between them just to make sure that the bot is right about it, but all the other intents that we’re accepting for the Morrie’s bot really don’t sound like oil change. We’re pretty confident that we’ll get that right more often than not.
Brian Morin: A question came in here and said, you shared voice examples not chat examples. You’re right. That’s true. You can go to our website. We have plenty to showcase of what we do as far as supporting digital virtual agents. We support the latest enrich web chat technology, and if you go there, you’ll see plenty of experiences showcase and you can see kind of mirrored even side by side, what does an interaction sound like when it’s done over voice as it’s done in parallel with chat. Mark, some questions, a couple of questions came in, some good questions I thought came in when you were giving the demo. One here is I see he’s listening. It made him reflect on the personal experience he has had where AI repeats back the option that he’s selected to make sure that he had it right, like the example, I heard oil change, is that correct. He wanted your thoughts on, what are your thoughts on this playback that it takes more of the customer’s time to do it on one side, but on the other side it helps, I guess you assure him or her that they were heard correctly.
Mark Landry: Yeah, that’s along the lines of the question I just answered. To give you another example of that, the chart that Marilyn showed where we’re taking a hotel reservation. In the original version of that, that the client had wanted us to implement, they had us asking, first of all, way too many questions, but also repeating back the answer and confirming it every single time. So it was doubling the experience and how long it took for the caller. That’s just ultimately the worst. Callers hate that. What we do is we just focus on those questions that we know we need to get a confirmation on. Those are the ones we do, and the rest of them we don’t. We understand if the data coming back that we’re getting this intent correct, oil change, correct, 90% something of the time, then we don’t need to ask a confirmation on that. It’s all about just iterating, testing it out and doing what we’re confident we know.
Brian Morin: A number of questions came in related to the text to speech. Wanted to know, was this recorded by a particular SmartAction voice talent? Can we make selections between your different voices, male and female voices? Mark.
Mark Landry: Yeah. Currently, we have one talent voice. The optimal versions are examples that we showed you. Our voice talent, our in-house voice talent, and she’s amazing. She’s recorded tens of thousands of street names, people names, all sorts of dynamic data, plus the prompts that we have.
Brian Morin: The takeaway being that otherwise dynamic data from other TTS models that can sound a little robotic by doing all that extra work, it makes it sound human sounding as the audience heard.
Mark Landry: That’s correct. Now, TTS engines are advancing at a rapid rate and they’re starting to sound more and more human. It is on our roadmap. We are keeping our finger on the pulse of that, and once we do arrive at one that is really, they can’t tell the difference, then we would switch. Then at that point, we will have the ability to change gender, all sorts of tweaks that we can do to change the character of voice.
Brian Morin: We like some of the voices that we hear that coming from Google and coming from Amazon, but we just don’t like them as well as our voice because we can do a thoroughly human sounding voice all the way through.
Mark Landry: That’s right.
Brian Morin: Marilyn and Mark, as you were scanning through some of these, any of these jump out to you that we want to bubble up for the rest of the audience.
Marilyn Cassedy: I’ll tag onto the voice talent topic actually because one of the things that I think is really interesting about working with a voice actress is actually that we’re able to change the tone a little bit in her thoughts or her approach to a conversation more than we are with the TTS. Even the best TTS voices today, I don’t think that you can change it to be a little bit bubbly or if we’re in kind of more of a sales mode or a little bit sterner if we might be making a collection call. Working with voice talent directly allows us to kind of make sure that the emotion you want the customer to feel through the phone call is more available to us, but I think otherwise Mark did a great job explaining that one.
Mark Landry: No, that’s really a good point. She’s a good actress and she takes direction well and understands context. She can change her character or her motivation to fit that context as you said. What happens if something happens to your voice valent? I love that question. I get that question a lot.
Brian Morin: Do you really?
Mark Landry: Yeah. Thank you, Esther. This goes along to our roadmapping conversation where I can’t tell too much about what we’re doing in the back of the house in terms of R and D, but we are seeing a future at SmartAction where an automated TTS voice is indistinguishable from the human voice talent that we have. Pretty soon we’ll not have to be worried about that other than just worried about her safety as a genuine human emotion. Another question… Thanks, Esther. Another question from Mary, am I too much of a native New Englander that I find the VA too slow? That’s also a good question. We don’t get that one a lot though because we used to have someone, and Marilyn’s good friends with this person that used to work with us, Liam. He’s from New York and he spoke so fast.
He’s a smart guy and he just speaks so quickly. It wouldn’t cause any problems in our conversations obviously, but if our voice, I used to tell him, if our voice bots spoke as quickly as he did, we’d have a lot of people pressing operator because we have to design these things for not the lowest common denominator, but basically the median so enough people have to be able to follow, but especially if we’re doing something like… We have a bot that does utility in an area of Florida where mostly it’s a retirement population. It’s elderly people. We have a lot of medical bots that service the same population type. We definitely pull that speed back and speak slowly and clearly. In other situations where we want to do a sales call or something like that, we’ll pep it up to ramp the excitement up. We have those levers with the human voice actor, but we don’t want to go too fast that we risk losing callers.
Brian Morin: Some of it too is what we go through in our iterative process on negotiating the length of time that the AI is waiting to respond back. We want this to operate as close to the speed of conversation as we can possibly get it. We’re highly frustrated when we try other solutions that seem to be painfully, painfully slow in speed of conversation.
Mark Landry: They’re from the South. I’ve had to speed it up since I moved to LA.
Brian Morin: Isabella has a good question here. She appreciates the pizza and the lunch, but she wants to understand can this understand more complex natural language? Now, this is an interesting conversation, Isabella, as we get into it because there is a real ebb and flow between how complex do we really want this conversation to be in natural language because keep in mind you are doing this over telephony, and the more complex or long that your sentences are in getting a response from somebody, the likelihood of you’ve being able to narrow the aperture down to what the intent really is begins to lower. Even though yes, we can design things to be more complex in nature from a natural language standpoint, we’re always trying to design things in such a way to really narrow the aperture of the response so we can make sure that we’re sharing the highest accuracy possible.
A really good example would be FAQ. Do you see anybody doing FAQ over voice? You don’t see FAQ over voice because that would be a highly complex natural language interaction to do. You wouldn’t get as high of a rater’s response back on accuracy. So if there’s an FAQ, we would never put that in voice, but we would put FAQ in chat and we would always send users to a chat for FAQ because we can run a very accurate NLP engine against that in chat and not have to worry about the speech rec side. Another question here, this is on how do we do with accents and dialects? We do really well with accents and dialects. We think that we do as well as in many cases humans do with accents and dialects. One of the pieces that our model is trained on is all the speeches given to NATO over the years as part of our training set. We have examples. If you go to our website, if you go to smartaction.ai/listen, we do have some examples of what that sounds like with accents and dialects. Any other color on that, Marilyn or Mark?
Mark Landry: No, that was very well said. Thank you.
Marilyn Cassedy: I would say that even back to the same point as your point about complexity again, understanding accents over telephone lines is a hard, hard thing to do, and so I would definitely put a sort of best in class in our ability to do so, but it’s always going to be a challenging problem just with that reduced resolution on the audio files.
Brian Morin: Yup. This is a pretty good question here. Mark, I’ll take a stab at this and then Mark or Marilyn, you’ll come in and play clean up on it as it says, as we’ve seen companies transition conversational AI, what are the common or typical challenges for first time users? Now, the first thing that would come to my mind is that sometimes we’ll end up in a situation where an organization is trying to make that transition in terms of what they could obtain from an ROI standpoint. Obviously, that’s the compelling event for AI is the ROI, but sometimes it gives the… Organizations come in with the idea that they want to automate everything irrespective of CX when in fact you don’t want to automate everything. You only want to automate the interactions where or believe that AI will perform as better, as well or better than a live agent.
Now, this doesn’t mean that you have to draw these clean buckets of interactions for AI where these interactions go to humans, these interactions go to AI. What we see with most clients that we work with is really a symbiotic relationship between AI and humans even within the same interactions where we ask ourselves the question, how much of this interaction should belong with AI or start with AI, and when and for what reasons should it be transferred to a human, either to finish a call or to handle the exclusions that can occur in the conversation? Let me give you a really good example of this is that we have an interaction that we automate and it has to do with getting a claim approval from State Farm where body shops will call in with State Farm policy numbers, and there are 17 back and forth turns in that conversation.
That’s a really complex conversation. There’s many different paths someone can take with that many back and forth. The client initially thought this is too complex to even automate it all, but as we dug deeper, we were able to define what we will call the happy path that the majority of callers take and determine that 70% of your callers are taking what we will call a happy path. The idea being is that you can use AI for the happy path, and that anytime there’s an exclusion that does occur during that back and forth, will you flip those exclusions to live agents. So that might be a more extreme example, but we see that example across many, many interactions that we support.
Mark Landry: Yeah. If you’re talking about the end user being the caller, when we’re first implementing a new AI experience for a client and their customers hear for the first time what would be the biggest challenges for the customers calling in. Really, we don’t see an issue unless it’s a situation where the customer is used to speaking to a human. A lot of customers don’t call habitually into one customer service line. They’ll have a one-off thing they need to do, and if it happens to be a robot that they’re getting, answering the phone, they don’t know a difference because they’re not used to speaking to someone. If it’s a situation where the caller habitually does call in to speak to someone to reorder their medication or whatever, there’s a little bit of a, let me see how this… It’s like, let me see if this robot is smart enough to handle what I want to do. The way we prove it is that if we know the person’s account, we read them by name and predict by their data what they’re calling for. If they just ordered something, are you calling about your recent order. Well, yeah, I am. I mean, this thing understands me.
Marilyn Cassedy: The Morrie’s example, are you calling about your upcoming appointment? You have an appointment later this week, so 80% of the time I bet you’re calling about that appointment, but 20% of the time you’ve got a second issue. You’ve got two different Cadillacs. Lucky guy, right?
Mark Landry: There’s some things that people don’t want to talk to a human about. I don’t want to tell you why I want to make a doctor’s appointment, I just want to make the appointment. I’ll tell a robot because it’s not going to tell anybody or think anything about it. It’s not going to judge me. I don’t want to talk to a human about my financial situation or collections. A robot, it’s fine. It’s not going to judge me. It’s not going to say I’m a deadbeat or bad person for not paying my bill and I can negotiate the payment with the robot without having any sort of angst about that.
Brian Morin: Well, we’re up here near the top of the hour. It looks like most of our audience is actually hung on for the whole thing, so we appreciate your time and attention and hope that it was worth the mind share. We will be sending over a copy of the deck and the on-demand tomorrow. Again, the info at SmartAction on screen if you would like to any other answers to questions or some type of engagement. With that said, Mark, Marilyn, maybe Marilyn, we’ll start with you. Any partying words?
Marilyn Cassedy: Yeah, I think the takeaway I would take from this is just to think at this level around what do you need to do to make sure that you’re successful with an implementation like this? It begins with picking the right speech recognition and to doing the right design and making sure that you’re working with a vendor who’s going to be with you through that tuning journey to optimize your application’s performance.
Mark Landry: Then I would say to piggyback on that, you’re either going to build it yourself and have to do all of what we do on your own or go with SmartAction that does it as a service.
Brian Morin: Okay. Well, I don’t think I could say it any better than myself. I hope this was informative, and of course we look forward to any ongoing and future conversations. I hope everyone has a great rest of your day. Marilyn, Mark, thank you.
Marilyn Cassedy: Thanks.
Brian Morin: Thank you.